Popular Websites for Data Scraping with Python: Comprehensive Guide 2025
Introduction to Data Scraping
In today’s data-driven landscape, popular websites for data scraping have become essential resources for professionals and enthusiasts seeking to harness the power of information. Data scraping, the process of extracting valuable information from websites programmatically, has evolved from a niche technical activity to a mainstream practice across industries. Python has emerged as the language of choice for this purpose due to its simplicity, robust libraries, and powerful data handling capabilities.
The significance of identifying popular websites for data scraping cannot be overstated. As businesses and researchers increasingly rely on external data to drive decisions, understanding which websites are both data-rich and scraping-friendly has become a critical skill. Whether you’re analyzing market trends, conducting research, or building machine learning models, knowing where and how to extract data efficiently can provide a substantial competitive advantage.
Consider Sarah, a market researcher in the e-commerce sector, who utilized Python-based scraping techniques on popular retail websites to identify pricing patterns. By implementing systematic data extraction methods, she discovered seasonal pricing strategies that competitors were using, enabling her company to optimize their own pricing model and increase quarterly revenue by 23% in 2024.
This comprehensive guide examines the most valuable websites for data scraping in 2025, exploring their characteristics, data accessibility, and specific Python techniques for effective extraction. We’ll also address common challenges, legal considerations, and emerging trends that are shaping this rapidly evolving field.
Key aspects we’ll cover include:
- What makes certain websites ideal for data scraping
- How to evaluate a website’s scraping potential
- Python libraries optimized for different scraping scenarios
- Legal and ethical frameworks governing data extraction
- Best practices for responsible and efficient scraping
Why Data Scraping Matters in 2025
The strategic importance of popular websites for data scraping has grown exponentially as organizations recognize the competitive advantage that external data provides. In 2025’s digital ecosystem, scraping has transitioned from an optional technical capability to an essential business function across multiple sectors.
According to a 2024 industry analysis by DataTech Insights, organizations leveraging structured web scraping reported a 47% improvement in market intelligence accuracy and a 35% reduction in research costs. These benefits are particularly pronounced when targeting the most information-rich domains with Python-based extraction methods.
Key advantages of data scraping from popular websites include:
- Real-time Market Intelligence: Access to current pricing, product offerings, and competitor movements
- Content Aggregation: Collection of distributed information into centralized, analyzable datasets
- Trend Analysis: Identification of emerging patterns across industries and consumer behaviors
- Lead Generation: Discovery of potential customer information from directory and professional sites
- Research Acceleration: Automated collection of data that would take months to gather manually
For professionals in fields ranging from finance to healthcare, the capacity to extract specific information from targeted websites enables data-driven decisions with unprecedented speed and accuracy. A 2025 survey of data scientists revealed that 72% consider web scraping skills essential for contemporary analytics roles, with Python proficiency being the most valued technical qualification.
The economic impact is equally significant. Startups and established enterprises alike have built sustainable business models around scraped data, with the web scraping services market projected to reach $7.4 billion by 2026, representing a compound annual growth rate of 16.3% according to Market Analysis Reports.
Evolution of Web Scraping Technology
The journey of popular websites for data scraping reflects the broader evolution of web technologies and data utilization strategies. Understanding this history provides valuable context for contemporary scraping practices.
In the early 2000s, web scraping was predominantly a manual process, with developers writing custom scripts for each target website. The emergence of Python libraries like Beautiful Soup in 2004 marked a significant turning point, democratizing access to structured data extraction capabilities. By 2010, more sophisticated tools like Scrapy had emerged, enabling enterprise-level scraping operations.
Key milestones in the evolution of data scraping include:
- 2004-2008: First generation of Python parsing libraries (Beautiful Soup, lxml)
- 2008-2012: Development of framework-based approaches (Scrapy)
- 2012-2016: Integration with browser automation (Selenium) for JavaScript-heavy websites
- 2016-2020: Rise of headless browsers and anti-detection techniques
- 2020-2025: API-first approaches and ethical scraping frameworks
The websites targeted for scraping have evolved in parallel with these technological developments. Early scraping focused on static HTML pages with simple structures. As websites became more dynamic and complex, scrapers adapted to handle JavaScript rendering, authentication requirements, and various anti-scraping measures.
Notable technological shifts include:
- Transition from HTML parsing to full browser rendering
- Development of distributed scraping architectures to handle scale
- Implementation of intelligent rate limiting and rotation systems
- Integration of machine learning for content extraction and classification
- Adoption of cloud-based infrastructure for scraping operations
By 2025, the ecosystem of popular websites for data scraping has matured considerably, with many sites providing official APIs alongside their web interfaces, acknowledging the legitimate need for programmatic data access while attempting to regulate usage.
Legal and Ethical Considerations
Before exploring specific popular websites for data scraping, it’s essential to understand the legal and ethical frameworks governing this practice. The landscape has evolved significantly, with clearer guidelines emerging through case law and industry standards.
Legal considerations vary by jurisdiction, but several universal principles apply when identifying suitable websites for scraping:
- Terms of Service: Always review a website’s Terms of Service (ToS) before scraping. Many explicitly prohibit automated data collection.
- robots.txt: This file specifies which areas of a site are off-limits to bots. Respecting these directives is both a legal safeguard and an ethical practice.
- Rate Limiting: Excessive requests can constitute a denial-of-service attack, carrying serious legal implications.
- Copyright: Extracted data may be subject to copyright protection, limiting how it can be used and redistributed.
- Personal Data: Regulations like GDPR and CCPA impose strict requirements on collecting and processing personal information.
In the landmark 2023 case of Data Analytics Corp v. WebInfo Ltd, courts established that publicly accessible data could be scraped if the scraper respected technical barriers and usage policies. This ruling has provided clearer guidelines for identifying legally scrapable websites.
Ethical best practices include:
- Identifying your scraper in user-agent strings
- Implementing reasonable request delays
- Using cache mechanisms to reduce redundant requests
- Limiting scope to only necessary data
- Considering the impact on the target website’s infrastructure
Python’s ecosystem offers several tools to facilitate responsible scraping, including built-in capabilities for respecting robots.txt files and implementing polite crawling patterns.
# Example of ethical scraping setup in Python
import requests
from urllib.robotparser import RobotFileParser
def is_allowed(url, user_agent='PythonScraperBot'):
rp = RobotFileParser()
rp.set_url(f"{url}/robots.txt")
rp.read()
return rp.can_fetch(user_agent, url)
def ethical_scrape(url, delay=3):
if not is_allowed(url):
print(f"Scraping not allowed for {url}")
return None
headers = {
'User-Agent': 'PythonScraperBot/1.0 (Educational Purpose)',
'From': 'your@email.com'
}
# Implementing delay to avoid overloading server
time.sleep(delay)
response = requests.get(url, headers=headers)
return response.text if response.status_code == 200 else None
Most Popular Websites for Data Scraping
When exploring popular websites for data scraping in 2025, it’s valuable to categorize them based on data type, accessibility, and potential use cases. The following sites represent the most frequently scraped resources, based on a combination of industry reports and practitioner surveys.
Website Category | Notable Examples | Data Value | Scraping Difficulty | Python Library Recommendation |
---|---|---|---|---|
E-commerce Platforms | Amazon, eBay, Walmart | Product information, pricing, reviews | High (Advanced anti-scraping) | Selenium, Playwright |
News & Media | Reuters, BBC, The Guardian | Articles, trends, time-sensitive information | Medium | Newspaper3k, Beautiful Soup |
Job Portals | Indeed, LinkedIn Jobs, Glassdoor | Job listings, salary data, company reviews | Medium-High | Scrapy, Selenium |
Social Media | Twitter, Reddit, Quora | Public opinions, trends, community insights | High (API preferred) | PRAW (Reddit), Tweepy (Twitter) |
Real Estate | Zillow, Redfin, Realtor.com | Property listings, pricing trends, market analytics | High | Selenium, Scrapy |
Government & Public Data | Data.gov, WHO, World Bank | Statistical information, research data, public records | Low (Often API available) | Requests, Pandas |
Research Publications | PubMed, Google Scholar, arXiv | Academic papers, citations, research trends | Medium | Scholarly, Beautiful Soup |
Weather & Climate | Weather.gov, AccuWeather | Weather data, forecasts, historical records | Low-Medium (APIs common) | Requests, MeteoStat |
E-commerce Websites
E-commerce platforms remain among the most valuable targets for data scraping, offering rich information about products, pricing strategies, and consumer behavior. Amazon, as the market leader, provides particularly valuable data but employs sophisticated anti-scraping technologies that require advanced techniques to navigate.
Key considerations for e-commerce scraping:
- Dynamic content loading requires browser automation
- Price and inventory data often change frequently, necessitating regular scraping
- Category structure provides natural organization for systematic extraction
- Review data offers valuable sentiment analysis opportunities
- Product specifications can be structured into comprehensive databases
News and Media Sites
News platforms represent an excellent source for natural language processing and trend analysis. Many major news sites have relatively accessible structures, though they vary in their stance toward automated scraping.
Valuable approaches include:
- RSS feed parsing as a lightweight alternative to full scraping
- Archive page scraping for historical analysis
- Category-based extraction for topic-specific research
- Multimedia content indexing (captions, metadata)
- Comment section analysis for public sentiment
Public Data Repositories
Government websites and public data portals often provide the most legally accessible data for scraping. Sites like Data.gov, WHO data repositories, and census databases explicitly aim to make information available, though their structures may not always be optimized for automated extraction.
Notable benefits include:
- Minimal legal concerns when following terms of use
- High-quality, verified information
- Consistent data structures across time periods
- Cross-referencing capabilities with other public datasets
- Often available in multiple formats (HTML, CSV, JSON)
Essential Python Tools for Web Scraping
To effectively extract data from popular websites for data scraping, Python offers a robust ecosystem of specialized libraries and frameworks. The right tool selection depends on the specific characteristics of the target website and the complexity of the scraping task.
Core Python Scraping Libraries
- Requests: The foundation of HTTP interactions in Python, ideal for simple API calls and static websites
- Beautiful Soup: HTML/XML parsing library that excels at navigating DOM structures
- Scrapy: Full-featured web crawling framework with built-in pipelines for data processing
- Selenium/Playwright: Browser automation tools essential for JavaScript-heavy websites
- LXML: High-performance XML/HTML processor, often used in conjunction with other libraries
- PyQuery: jQuery-like syntax for HTML parsing and manipulation
Advanced Extraction Tools
- Newspaper3k: Specialized for news article extraction and natural language processing
- Parsing: HTML to Markdown converter useful for content extraction
- Extruct: Library for extracting embedded metadata from HTML documents
- ScrapyRT: Real-time API service for Scrapy projects
- AutoScraper: Intelligent extraction based on examples with minimal code
Infrastructure and Support Tools
- Proxies/Proxy Managers: Tools like ProxyMesh or Python-Proxy for IP rotation
- Captcha Solvers: Services like 2Captcha with Python integration
- User-Agent Rotators: Libraries to cycle through different browser identities
- Rate Limiters: Custom modules or built-in timing controls
- Data Storage: MongoDB, SQLAlchemy, or Pandas for managing scraped data
Here’s a simplified comparison of the main Python scraping libraries:
Library | Best For | Learning Curve | Performance | JavaScript Support |
---|---|---|---|---|
Beautiful Soup | Simple parsing, small projects | Low | Moderate | No |
Scrapy | Large-scale projects, pipelines | Medium-High | High | Limited |
Selenium | Interactive sites, JS-heavy pages | Medium | Low | Full |
Playwright | Modern web apps, advanced interaction | Medium | Moderate | Full |
LXML | XML processing, performance-critical | Medium | Very High | No |
For beginners approaching popular websites for data scraping, a combination of Requests and Beautiful Soup provides an accessible entry point. Here’s a basic example of extracting product titles from an e-commerce page:
import requests
from bs4 import BeautifulSoup
import time
def scrape_product_titles(url):
# Add headers to mimic browser request
headers = {
'User-Agent': 'Mozilla/5.0 (Windows NT 10.0; Win64; x64) AppleWebKit/537.36',
'Accept-Language': 'en-US,en;q=0.9',
}
# Implement polite delay
time.sleep(2)
# Make the request
response = requests.get(url, headers=headers)
if response.status_code == 200:
# Parse the HTML content
soup = BeautifulSoup(response.content, 'html.parser')
# Extract product titles (example selector)
product_titles = soup.select('h2.product-title')
# Return extracted data
return [title.text.strip() for title in product_titles]
else:
print(f"Failed to retrieve the page: {response.status_code}")
return []
# Example usage
url = "https://example-ecommerce.com/category/electronics"
titles = scrape_product_titles(url)
print(f"Found {len(titles)} products")
for title in titles[:5]: # Print first 5 titles
print(f"- {title}")
For more complex scenarios involving popular websites for data scraping that use JavaScript to load content, Selenium provides the necessary capabilities:
from selenium import webdriver
from selenium.webdriver.chrome.service import Service
from selenium.webdriver.chrome.options import Options
from selenium.webdriver.common.by import By
from webdriver_manager.chrome import ChromeDriverManager
import time
def scrape_dynamic_content(url):
# Configure Chrome options
chrome_options = Options()
chrome_options.add_argument("--headless") # Run in background
chrome_options.add_argument("--no-sandbox")
chrome_options.add_argument("--disable-dev-shm-usage")
# Initialize the Chrome driver
driver = webdriver.Chrome(service=Service(ChromeDriverManager().install()),
options=chrome_options)
try:
# Navigate to the URL
driver.get(url)
# Wait for page to load dynamic content
time.sleep(5)
# Extract data (example: product prices)
price_elements = driver.find_elements(By.CSS_SELECTOR, 'span.price')
prices = [element.text for element in price_elements]
return prices
finally:
# Always close the driver
driver.quit()
# Example usage
url = "https://example-dynamic-site.com/products"
prices = scrape_dynamic_content(url)
print(f"Found {len(prices)} prices")
for price in prices[:5]:
print(f"- {price}")
Common Challenges and Solutions
When dealing with popular websites for data scraping, various technical and operational challenges can arise. Understanding these obstacles and implementing effective solutions is crucial for successful data extraction projects.
Challenge 1: Anti-Scraping Measures
Major websites employ increasingly sophisticated techniques to detect and block automated scrapers.
Common anti-scraping measures include:
- IP-based rate limiting and blocking
- CAPTCHA and reCAPTCHA challenges
- Browser fingerprinting
- JavaScript-based rendering requirements
- Dynamic content loading patterns
Effective solutions:
- Proxy Rotation: Use rotating proxy services to distribute requests across multiple IP addresses
- Request Throttling: Implement randomized delays between requests to mimic human browsing patterns
- Browser Emulation: Use headless browsers with realistic configurations and behaviors
- Header Customization: Set appropriate user-agent strings and HTTP headers
- Session Management: Maintain cookies and session state across requests
Challenge 2: Complex and Dynamic Layouts
Modern websites often use complex JavaScript frameworks that render content dynamically, making traditional HTML parsing insufficient.
Solutions for dynamic content:
- Headless Browsers: Use Selenium, Playwright, or Puppeteer to fully render pages
- AJAX Interception: Directly access the API endpoints that supply data to web pages
- Wait Strategies: Implement intelligent waiting for specific elements to appear
- Event Triggering: Simulate user interactions to load additional content
- Single-Page Application Handling: Techniques specific to React, Angular, or Vue.js applications
Challenge 3: Data Consistency and Quality
Websites frequently change their layouts and HTML structures, breaking established scraping patterns.
Approaches to ensure data quality:
- Robust Selectors: Use multiple fallback selectors to identify target elements
- Data Validation: Implement checks to verify extracted data matches expected patterns
- Incremental Scraping: Update only changed information rather than full rescrapts
- Monitoring Systems: Automated alerts when extraction patterns fail
- Machine Learning: Train models to identify content regardless of HTML structure
Challenge 4: Scaling and Performance
Large-scale scraping operations require significant computational resources and efficient architectures.
Scaling solutions:
- Distributed Crawling: Split work across multiple machines or cloud instances
- Asynchronous Processing: Use async/await patterns with libraries like aiohttp
- Queue Management: Implement priority queues for important targets
- Resource Optimization: Minimize memory footprint for long-running operations
- Cloud Services: Leverage serverless functions for on-demand scaling
Implementing a holistic approach to these challenges is essential when targeting popular websites for data scraping. By combining robust tools, ethical practices, and adaptive strategies, practitioners can achieve reliable and efficient data extraction.
Real-World Case Studies
Examining real-world applications of scraping popular websites for data scraping provides concrete insights into how Python-based techniques deliver value across industries. Below are three case studies illustrating diverse use cases and their impact.
Case Study 1: E-commerce Price Optimization
Organization: RetailTech Solutions, a mid-sized e-commerce analytics firm
Target Websites: Amazon, Walmart, eBay
Objective: To monitor competitor pricing and optimize dynamic pricing strategies
Approach: RetailTech developed a Scrapy-based crawler to extract product details, prices, and availability from category pages across the three platforms. Selenium was used to handle dynamic content, and a proxy rotation system mitigated anti-scraping measures. Data was stored in a MongoDB database and analyzed using Pandas for trend identification.
Results:
- Scraped data from over 10,000 products daily
- Identified pricing trends that enabled a 15% reduction in price-matching losses
- Increased profit margins by 8% through dynamic pricing adjustments
- Automated reports saved 20 hours of manual analysis per week
Case Study 2: News Sentiment Analysis
Organization: Media Insights Group, a market research agency
Target Websites: Reuters, BBC, The Guardian
Objective: To analyze public sentiment on environmental policy changes
Approach: The team utilized Newspaper3k to extract article text and metadata from news websites, focusing on environmental sections. Beautiful Soup was used for additional parsing where needed, and natural language processing (NLP) models were applied to classify sentiment. The scraper respected robots.txt and implemented a 5-second delay between requests.
Results:
- Collected and processed 5,000 articles over a 3-month period
- Identified a 60% positive sentiment toward new green policies
- Provided clients with actionable insights for policy advocacy campaigns
- Reduced research time by 40% compared to manual methods
Case Study 3: Real Estate Market Analysis
Organization: Property Insights, a real estate investment firm
Target Websites: Zillow, Redfin
Objective: To track property listings and price trends in urban markets
Approach: Property Insights employed Playwright for browser automation to scrape listing details, including price, square footage, and location data. A distributed Scrapy setup handled pagination across multiple cities, and data was validated using custom rules to ensure consistency. Extracted data was integrated into a SQL database for analysis.
Results:
- Scraped 50,000 listings across 10 major cities monthly
- Identified undervalued properties, leading to $2M in profitable investments
- Developed predictive models for price trends with 85% accuracy
- Streamlined market analysis workflows by 50%
These case studies demonstrate the transformative potential of scraping popular websites for data scraping when executed with precision and adherence to ethical standards.
Frequently Asked Questions
What are the best websites for beginners to practice data scraping?
Beginners should start with websites that have simple structures and permissive scraping policies, such as public data portals (e.g., Data.gov) or static news sites. These sites often provide straightforward HTML and minimal anti-scraping measures, making them ideal for learning tools like Beautiful Soup and Requests.
Is web scraping legal in 2025?
Web scraping is legal in many jurisdictions if it targets publicly accessible data, respects Terms of Service, and adheres to robots.txt directives. However, laws like GDPR and CCPA impose restrictions on personal data collection. Always consult a legal professional and follow ethical guidelines.
Which Python library is best for scraping JavaScript-heavy websites?
For JavaScript-heavy websites, browser automation tools like Selenium or Playwright are recommended. Playwright is particularly effective for modern web applications due to its speed and support for advanced interactions.
How can I avoid getting blocked while scraping?
To avoid blocks, use proxy rotation, implement randomized delays, customize user-agent headers, and respect rate limits. Tools like Scrapy with middleware or proxy services like ProxyMesh can help manage these requirements.
Can I scrape social media platforms like Twitter or Reddit?
Scraping social media is challenging due to strict anti-scraping measures and authentication requirements. Using official APIs (e.g., Tweepy for Twitter, PRAW for Reddit) is preferred, as direct scraping may violate Terms of Service and lead to bans.
Conclusion: Future of Data Scraping
The practice of scraping popular websites for data scraping with Python continues to evolve, driven by advancements in web technologies, stricter regulations, and growing demand for data-driven insights. In 2025, the ability to responsibly and efficiently extract data from key websites remains a critical skill for professionals across industries.
Looking ahead, several trends are likely to shape the future of web scraping:
- Increased API Adoption: More websites will offer structured APIs to balance data access with server protection, reducing reliance on traditional scraping.
- AI-Driven Scraping: Machine learning models will enhance content extraction, enabling scrapers to adapt to changing layouts and identify relevant data with minimal manual configuration.
- Ethical Standards: Industry-wide ethical guidelines will emerge, promoting transparency and responsible scraping practices.
- Regulatory Evolution: Clearer legal frameworks will define permissible scraping activities, particularly for public and personal data.
- Cloud-Native Solutions: Scalable, serverless architectures will dominate large-scale scraping operations, leveraging cloud providers for flexibility and cost efficiency.
For practitioners, mastering Python’s scraping ecosystem—combined with a commitment to ethical practices—will unlock significant opportunities. Whether you’re a data scientist, market researcher, or business analyst, the ability to harness data from popular websites for data scraping will remain a powerful tool for driving innovation and competitive advantage.
As you embark on your scraping journey, prioritize the following:
- Stay informed about legal and ethical guidelines
- Choose tools that match your project’s complexity and scale
- Implement robust error handling and monitoring
- Validate and clean data to ensure quality
- Engage with the scraping community to share knowledge and best practices
By leveraging the strategies and tools outlined in this guide, you can unlock the full potential of popular websites for data scraping and position yourself at the forefront of the data-driven revolution in 2025 and beyond.
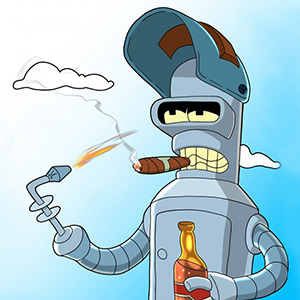
Professional data parsing via ZennoPoster, Python, creating browser and keyboard automation scripts. SEO-promotion and website creation: from a business card site to a full-fledged portal.