7 Game-Changing Ways Streamlit Applications Boost Your Data Projects
Streamlit Applications have redefined how data enthusiasts, developers, and professionals transform raw numbers into interactive experiences. Whether you’re a hobbyist tinkering with datasets or a seasoned coder building client-facing tools, this powerful framework delivers simplicity without sacrificing depth. Imagine crafting a web app in hours, not weeks—sounds tempting, right? This article dives into expert insights, offering practical tips tailored to those eager to elevate their projects with minimal fuss.
Unlike traditional methods bogged down by complex setups, Streamlit strips away the clutter. Its Python-based ecosystem lets you focus on what matters: turning ideas into reality. From visualizing trends to deploying dashboards, the possibilities stretch as far as your curiosity takes you. Let’s explore how this tool reshapes workflows and unlocks creative potential.
What Makes Streamlit Applications Stand Out?
Streamlit isn’t just another library—it’s a launchpad for rapid development. Built with Python lovers in mind, it bridges the gap between data science and web interfaces. You write code, hit run, and voilà—an app appears, ready to impress. No need to wrestle with JavaScript or HTML unless you want to; Streamlit handles the heavy lifting behind the scenes.
Its secret sauce? Simplicity paired with flexibility. Developers can prototype ideas fast, tweaking visuals or adding interactivity on the fly. For enthusiasts, it’s a playground to experiment without drowning in documentation. Compared to alternatives like Flask or Dash, Streamlit cuts the learning curve while keeping robust features intact.
- Speed: Build apps in hours, not days.
- Ease: Python syntax you already know.
- Interactivity: Widgets that engage users effortlessly.
The Power of Python in Streamlit
Leveraging Python’s vast ecosystem, **Streamlit Applications** integrate seamlessly with libraries like Pandas, NumPy, and Matplotlib. This synergy empowers you to analyze data and present it in one smooth workflow. Picture this: pulling insights from a CSV, plotting them dynamically, and letting users filter results—all in a single script. It’s efficient, elegant, and downright satisfying.
For professionals, this means less time juggling tools and more time delivering value. Hobbyists, meanwhile, get to flex their skills without needing a computer science degree. The result? A tool that scales with your ambition, whether you’re mocking up a proof-of-concept or polishing a production-ready app.
How to Achieve Stunning Visuals with Streamlit Applications
Visuals can make or break a data project, and Streamlit excels at turning numbers into eye-catching displays. With built-in support for charts and graphs, crafting stunning dashboards becomes second nature. Pair it with libraries like Plotly or Altair, and you’ve got a recipe for dynamic, user-friendly designs that pop off the screen.
Start simple: a line chart showing sales trends. Add a slider, letting users zoom into specific years. Suddenly, your app isn’t just informative—it’s interactive. This hands-on approach hooks your audience, keeping them engaged longer. Plus, Streamlit’s real-time updates mean changes reflect instantly, saving you from endless refresh cycles.
Key Benefits of Streamlit’s Visualization Tools
Why choose Streamlit over clunky alternatives? Its visualization toolkit prioritizes usability without compromising power. You don’t need to be a design guru to create something polished—prebuilt components handle the grunt work. Meanwhile, customization options let you tweak colors, layouts, and interactivity to suit your vision.
Consider a scenario: you’re presenting data to stakeholders. A static PDF won’t cut it—they want to poke around, test assumptions. Streamlit delivers that flexibility, turning passive viewers into active explorers. It’s not just about looking good; it’s about making data actionable.
Feature | Benefit |
---|---|
Real-time updates | Instant feedback as users adjust inputs |
Library integration | Enhanced visuals with minimal code |
Deploying Your Streamlit App Like a Pro
Building an app is one thing; sharing it with the world is another. Streamlit’s deployment options make this step a breeze, even for newcomers. Platforms like Streamlit Community Cloud let you host apps for free, with GitHub integration streamlining the process. Push your code, and it’s live—simple as that.
For pros needing more control, Heroku or AWS offer scalable alternatives. These platforms handle traffic spikes and custom domains, perfect for client projects. Whichever route you pick, Streamlit ensures your app stays accessible, letting you focus on refining features instead of wrestling with servers.
Check out the official Streamlit documentation for step-by-step deployment guides.
Choosing the Right Hosting Platform
Streamlit Community Cloud suits quick demos and personal projects—zero cost, zero hassle. But what if you’re eyeing enterprise-grade reliability? Heroku’s flexibility shines, offering custom scaling and domain support. AWS, meanwhile, caters to heavy-duty needs with its vast infrastructure. Each option balances ease and power differently, so pick based on your goals.
Deploying isn’t the finish line—it’s the starting gun. Once live, gather feedback, tweak features, and watch your app evolve. Streamlit’s iterative nature makes this cycle smooth, keeping your project agile and audience-focused.
Unlocking Interactivity with Widgets
Interactivity transforms a good app into a great one. Streamlit’s widgets—sliders, buttons, dropdowns—bring your project to life with minimal effort. Want users to filter a dataset? Drop in a selectbox. Need real-time input? Add a text field. These tools empower your audience to explore data on their terms.
Take a machine learning demo: users tweak parameters, and the model updates instantly. It’s not just functional—it’s engaging. By handing control to your audience, you create an experience that sticks. No wonder developers rave about how Streamlit turns static scripts into dynamic conversations.
Customizing Widgets for Maximum Impact
Widgets aren’t one-size-fits-all—Streamlit lets you tailor them to your needs. Adjust ranges on sliders, populate dropdowns with dynamic data, or tie buttons to custom functions. This flexibility ensures your app feels intuitive, not cookie-cutter. Users notice the difference when controls align with their workflow.
Pro tip: keep it simple at first. Overloading an app with too many widgets can overwhelm users, diluting the experience. Start with essentials, test with real people, then scale up. The goal? Interactivity that enhances, not distracts.
Real-World Examples of Streamlit in Action
Need proof Streamlit delivers? Look at data scientists building portfolio dashboards. They pull stock prices with yfinance, plot trends with Plotly, and let users filter by ticker—all in under 100 lines of code. The result is a sleek, shareable tool that screams professionalism without breaking a sweat.
Or consider educators. A teacher whips up an app to simulate physics equations, sliders adjusting variables like gravity or mass. Students play, learn, and grasp concepts faster than any textbook could manage. Streamlit turns abstract ideas into tangible exploration, proving its worth across fields.
Case Study: Predictive Analytics Dashboard
Imagine a small business tracking sales forecasts. Using **Streamlit Applications**, a developer integrates a scikit-learn model, displays predictions with Altair charts, and adds filters for regions or products. Deployed on Heroku, it’s a client-ready solution in days—not months. That’s the kind of efficiency that turns heads.
The beauty lies in its adaptability. Swap sales for weather data, health metrics, or game stats—the framework bends to your needs. It’s less about what Streamlit does and more about what you can dream up with it.
Advanced Features to Level Up Your Apps
Ready to push boundaries? Streamlit’s advanced features—like caching and multipage apps—unlock next-level potential. Caching speeds up load times by storing computation results, perfect for heavy datasets. Multipage apps, meanwhile, organize complex projects into clean, navigable sections.
Picture a financial tool: one page for data input, another for analysis, a third for visualizations. Caching ensures recalculations don’t bog it down, while the layout keeps users oriented. These tweaks elevate your app from functional to phenomenal, impressing even picky critics.
For more on caching, explore this scikit-learn guide—it pairs beautifully with Streamlit’s ecosystem.
Integrating Machine Learning Models
Streamlit shines with machine learning. Load a trained model, add widgets for input, and display predictions in real time. A churn prediction app, for instance, lets users tweak customer data and see risks instantly. It’s a fast track from Jupyter notebooks to polished tools, no PhD required.
The trick? Keep models lightweight and preprocess data beforehand. Streamlit isn’t a compute beast—it’s a presentation layer. Offload heavy lifting to your training phase, and let the app focus on delivery. Done right, it’s a showcase of skill and smarts.
Troubleshooting Tips for Streamlit Success
Even the best tools hiccup. If your app lags, check for unoptimized loops or oversized datasets—caching often fixes this. Widgets misbehaving? Ensure variables update correctly with st.session_state. Streamlit’s community forums are goldmines for quick fixes, too.
Deployment woes hit hard sometimes. On Streamlit Cloud, a missing requirements.txt can crash your app—double-check dependencies. Heroku’s logs pinpoint errors fast, so lean on them. Troubleshooting isn’t glamorous, but mastering it keeps your project humming.
Common Pitfalls and How to Dodge Them
Newbies often overcomplicate layouts—resist the urge. Stick to Streamlit’s column and expander tools for clarity. Another trap? Ignoring mobile users. Test responsiveness early; a desktop-only app alienates half your audience. Small tweaks now save headaches later.
Documentation skimming bites back, too. Streamlit’s quirks—like widget state persistence—trip up rushed coders. Take an hour, read the fine print, and you’ll sidestep 90% of bugs. It’s less about genius and more about diligence.
Conclusion: Why Streamlit Applications Rewrite the Rules
Streamlit Applications don’t just streamline coding—they rethink what’s possible with data. By blending Python’s muscle with web-ready output, they hand you a superpower: turning insights into tools fast. Enthusiasts gain a sandbox to experiment; professionals get a shortcut to polished deliverables. It’s a rare mix of fun and function that keeps you coming back.
Reflect on this: every line of code you write with Streamlit builds not just an app, but a bridge—between ideas and impact, data and decisions. That’s the real win. So, grab a dataset, fire up your editor, and see where this journey takes you. The next breakthrough might just be a script away.
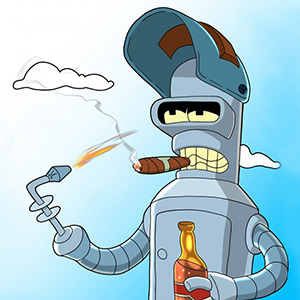
Professional data parsing via ZennoPoster, Python, creating browser and keyboard automation scripts. SEO-promotion and website creation: from a business card site to a full-fledged portal.