Scraping eBay – the largest e-commerce platforms in the world
With an endless stream of new listings and sales, the eBay marketplace doubles as a vast data portal for the enterprising. Millions of active listings contain a wealth of information – from pricing to demand signals, inventory status to seller behaviors. eBay‘s dominance as a global e-commerce platform also ensures scale and depth across categories lacking in smaller venues.
This abundance of open marketplace information waits to be tapped by those able to identify need and extract relevant data. For the innovative, possibilities span pushing pricing algorithms, devising demand-based inventory systems, identifying segment opportunities and underserved buyer needs, conducting competitor intelligence, predicting churn risk and countless other modeling and analysis applications. The benefit lies with those first able to connect questions with eBay’s data for timely and actionable market insights. Scraping eBay offers an automated way to extract information from listings at scale.
Why Scrape eBay Data
There are many potential applications for scraped eBay data:
- Competitive pricing research – Monitor competitor prices and inventory levels. Adjust pricing strategies accordingly.
- Market research – Analyze market demand, trends, and opportunities. Discover popular products and optimize inventory.
- Lead generation – Find contact information for eBay sellers. Reach out with marketing campaigns and partnership opportunities.
- Product research – Discover detailed product information like titles, descriptions, images, categories, shipping options, locations, etc. Useful for dropshipping or arbitrage.
- Reputation management – Monitor eBay feedback and reviews. Identify areas to improve the customer experience.
- eBay dropshipping – Automatically import eBay products to an ecommerce store. Sync pricing, inventory, images, etc.
Scraping provides a scalable way to quickly gather large amounts of eBay data compared to manual browsing or eBay APIs alone. The data can feed into business intelligence, machine learning, or process automation systems.
eBay Scraping Considerations
There are a few important factors to consider when parsing site:
- eBay Terms of Service – eBay forbids scraping without permission. However, scraping small amounts of data occasionally may be tolerated. Always consult eBay TOS before scraping.
- BOT detection – eBay actively tries to detect and block scraping bots. Proper proxies, headers, delays, captcha solving, and other evasion techniques are needed.
- Data accuracy – eBay listings change rapidly. The scraped data may become outdated quickly. Scheduling frequent scrapes can help reduce this issue.
- Spam prevention – When contacting eBay sellers, take measures to avoid being flagged as spam. Personalize messages, throttle outreach cadence, and monitor opt-out requests.
- Legal compliance – In some jurisdictions, scraping public data without permission can still be illegal. Seek legal counsel before scraping at scale.
Scraping eBay Listings
The first step in scraping eBay is identifying the target listings to extract data from. There are a few common approaches:
- Keyword search – Scrape listings matching a particular keyword or product name. Useful for monitoring specific items.
- Category scrape – Scrape all listings within a particular eBay category like electronics, fashion, motors, etc. Provides a broad market overview.
- Seller scrape – Scrape all current and historical listings from a particular eBay seller account. Helpful for competitor research.
- Auction monitoring – Continuously scrape newly listed items and bidding prices for timely arbitrage and auction sniping opportunities.
eBay provides an Advanced Search page that allows creating complex search queries to filter listings by parameters like price range, condition, product features, seller feedback score, shipping options, listing formats, and more. Crafting a focused search query helps scrape listings more efficiently compared to crawling across all of eBay.
Extracting Listing Data
Once the target eBay listings are identified, the key data points can be extracted. eBay listings contain a wealth of information:
- Title, description, and images
- Category and product identifiers
- Seller username and feedback score
- Number of views, watchers, and orders
- Start/end dates and listing duration
- Original listing price and current bid amount
- Buy it now option availability and price
- Shipping cost, options, and policies
- Item condition and return policies
- Item attributes like brand, model number, material, color, size, etc.
- Item location details like country, region, city, zip code
- Historical price data and bidding activity
This data can be extracted by parsing the HTML of the eBay listing pages. The key information is contained within HTML elements like <title>
, <meta>
, <span>
, <div>
, etc. An XPath or CSS selector is used to target the desired elements.
Structuring Scraped Data
To make the extracted eBay data actionable, it should be structured uniformly. For example, storing the data in a JSON dictionary or uploading it to a database table. Useful for downstream analysis and integration with other systems.
The data can be structured like:
{
"title": "New iPhone 11 Pro 256GB...",
"subtitle": "BRAND NEW,Factory Unlocked,FREE Delivery",
"description": "This iPhone is BRAND NEW, Factory Unlocked...",
"category": "Cell Phones & Smartphones",
"itemId": "2345534543",
"seller": "awesome-seller-9876",
"rating": 4.8,
"price": 799.99,
"shipping": 9.99,
"condition": "New",
"model": "iPhone 11 Pro",
"storage": "256GB",
"color": "Space Gray"
}
Proper data structuring allows easy querying, filtering, and aggregation. The scraped eBay data can then power everything from simple reporting dashboards to advanced machine learning pipelines.
Conclusion
Parsing platform opens up many possibilities to leverage eBay’s vast marketplace data at scale. With proper consideration around targeting, extraction, and structuring, scalable eBay scraping can become an invaluable business asset. As always, respect eBay’s terms of service and prioritize customer trust. Used ethically, scraped eBay data can unlock transformative business insights.
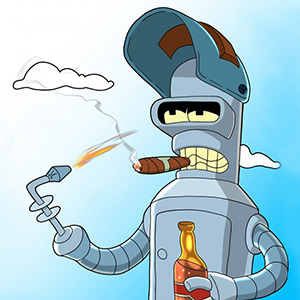
Professional data parsing via ZennoPoster, Python, creating browser and keyboard automation scripts. SEO-promotion and website creation: from a business card site to a full-fledged portal.