Scraping Amazon with Python: The Complete 2025 Guide
Introduction to Amazon Scraping
In today’s data-driven business landscape, scraping Amazon has become an essential practice for professionals and businesses looking to gain competitive insights, monitor pricing strategies, and make informed decisions. This comprehensive guide explores how Python has emerged as the premier tool for extracting valuable data from the world’s largest online marketplace.
Amazon’s vast product catalog contains millions of items with constantly changing prices, reviews, and availability information. For professionals ranging from e-commerce entrepreneurs to market researchers, manually tracking this information is virtually impossible. This is where scraping Amazon with Python becomes invaluable—automating the collection of targeted data at scale.
Consider the challenge faced by Michael, an e-commerce business owner who needed to monitor competitor pricing across hundreds of products. By implementing a Python-based Amazon scraping solution, he was able to automate price checks every four hours, allowing him to adjust his own pricing strategy dynamically and increase his profit margins by 18% within the first quarter of implementation.
This guide will take you through everything you need to know about scraping Amazon—from the fundamentals to advanced techniques, legal considerations, and practical implementation strategies. Whether you’re a Python developer looking to enhance your web scraping skills or a business professional seeking to leverage data for competitive advantage, you’ll find actionable insights and code examples to help you succeed in your Amazon scraping endeavors.
Why Amazon Scraping Matters
Scraping Amazon has become increasingly important for businesses and professionals worldwide as e-commerce continues to dominate retail markets. The strategic value of Amazon data extends far beyond simple price monitoring, offering insights that can transform business operations and strategy.
According to a 2024 industry analysis, companies utilizing automated Amazon data collection reported a 43% improvement in pricing strategy effectiveness and a 27% increase in conversion rates when they aligned their offerings with market trends identified through data analysis.
Key benefits of scraping Amazon include:
- Competitive Intelligence: Track competitor pricing, product launches, and promotional strategies
- Dynamic Pricing Optimization: Adjust your prices based on real-time market conditions
- Product Research: Identify trending products and untapped market opportunities
- Review Analysis: Understand consumer sentiment and product perception
- Inventory Management: Monitor stock levels and availability patterns
For manufacturers and brands, monitoring how their products are represented across Amazon’s marketplace has become critical. Sarah, a brand manager for a consumer electronics company, implemented a Python-based monitoring system that alerted her team whenever unauthorized sellers listed their products at prices that violated MAP (Minimum Advertised Price) policies. This system helped protect her brand’s reputation and maintain pricing integrity across all sales channels.
The significance of scraping Amazon will only increase as e-commerce continues to evolve, making it an essential skill for data analysts, e-commerce professionals, and market researchers in 2025 and beyond.
The Evolution of Amazon Scraping
The practice of scraping Amazon has undergone significant transformation since the early days of e-commerce. Understanding this evolution provides valuable context for current approaches and anticipates future developments in the field.
In the early 2010s, Amazon scraping was relatively straightforward, with simple HTML parsers sufficient for extracting basic product information. As the marketplace grew in complexity, so did the challenges of data extraction. By 2015, Amazon had implemented sophisticated anti-scraping measures, including dynamic page rendering, CAPTCHA systems, and IP-based rate limiting.
Key milestones in the evolution of Amazon scraping include:
- 2010-2013: Basic HTML parsing with libraries like Beautiful Soup was sufficient
- 2014-2016: Introduction of headless browsers to handle JavaScript-rendered content
- 2017-2019: Rise of specialized scraping tools and proxy networks to manage IP rotation
- 2020-2022: Integration of AI and machine learning for pattern recognition and CAPTCHA solving
- 2023-2025: Development of sophisticated stealth technologies and increased focus on ethical scraping practices
The Python ecosystem has evolved alongside these changes, with libraries like Scrapy, Selenium, and Playwright becoming increasingly powerful for handling complex scraping scenarios. The introduction of asynchronous libraries such as asyncio and aiohttp has significantly improved scraping efficiency, allowing for concurrent requests that maximize data collection while respecting rate limits.
Today’s Amazon scraping approaches prioritize ethical considerations, incorporating rate limiting, respectful crawling patterns, and compliance with legal requirements. This shift reflects a broader recognition that sustainable scraping practices benefit both data collectors and the platforms they interact with.
As we look ahead to the remainder of 2025 and beyond, we can expect further sophistication in both Amazon’s anti-scraping measures and the tools developed to ethically navigate them, creating an ongoing technological evolution in this space.
Practical Applications
Scraping Amazon extends far beyond academic interest, offering practical applications across numerous industries and business functions. These real-world use cases demonstrate the versatility and power of Python-based Amazon data extraction.
Price Monitoring and Competitive Analysis
Perhaps the most common application involves tracking competitor pricing strategies. E-commerce businesses use Amazon scrapers to monitor price fluctuations, promotional patterns, and discount strategies. This data feeds into dynamic pricing algorithms that automatically adjust product prices to maintain competitiveness while preserving profit margins.
Product Research and Market Analysis
Entrepreneurs and product developers leverage Amazon data to identify market gaps and trending products. By analyzing bestseller lists, customer reviews, and category rankings, businesses can make informed decisions about product development and inventory investments.
Jennifer, a product manager at a home goods company, used Python scripts to analyze Amazon reviews across competing products in her category. This analysis revealed specific features customers consistently mentioned as lacking, which her team incorporated into their next product iteration. The resulting product achieved a 4.7-star average rating upon launch, significantly outperforming competitors.
SEO and Content Optimization
Content marketers and SEO specialists analyze top-performing Amazon listings to understand successful keyword strategies, optimal title structures, and effective product descriptions. This intelligence helps optimize both Amazon listings and external e-commerce sites.
Review and Sentiment Analysis
Customer feedback contains invaluable insights about product strengths and weaknesses. By scraping Amazon reviews and applying natural language processing techniques, companies can quantify sentiment, identify common complaints, and track brand perception over time.
Market Trend Identification
Analyzing historical Amazon data reveals seasonal trends, emerging product categories, and shifting consumer preferences. This intelligence helps businesses anticipate market movements and position their inventory accordingly.
These applications demonstrate why scraping Amazon has become an essential practice for data-driven businesses across the e-commerce ecosystem. The ability to collect, analyze, and act upon this data creates significant competitive advantages in today’s fast-moving marketplace.
Challenges and Solutions
While scraping Amazon offers tremendous value, it presents significant technical and ethical challenges that must be navigated carefully. Understanding these obstacles and their solutions is essential for developing sustainable and effective scraping strategies.
Anti-Scraping Measures
Amazon employs sophisticated systems to detect and block automated data collection, including:
- CAPTCHA Challenges: Automated tests to distinguish humans from bots
- IP-Based Rate Limiting: Restricting the number of requests from a single IP address
- Dynamic Page Elements: Changing HTML structures and element IDs
- Behavioral Analysis: Detecting non-human browsing patterns
Solutions to Technical Challenges
Python developers have developed effective countermeasures to address these challenges:
Challenge | Solution | Implementation |
---|---|---|
IP Blocking | Proxy Rotation | Using services like Bright Data, Oxylabs, or rotating residential proxies |
CAPTCHA Detection | Headless Browsers | Selenium or Playwright with stealth plugins |
Dynamic Elements | Robust Selectors | XPath or CSS selectors with multiple fallback options |
Request Patterns | Human-like Behavior | Random delays, session maintenance, and realistic user agents |
Legal and Ethical Considerations
Beyond technical challenges, scraping Amazon involves navigating legal and ethical boundaries:
- Terms of Service Compliance: Amazon’s terms explicitly prohibit certain forms of automated data collection
- Copyright Issues: Product images and descriptions may be protected by copyright
- Rate Limiting: Ethical scraping requires respecting server resources
- Data Privacy: Personal information in reviews must be handled according to privacy regulations
Legal frameworks regarding web scraping continue to evolve, with landmark cases like hiQ Labs v. LinkedIn influencing the interpretation of computer access laws. While these cases don’t directly address Amazon scraping, they establish important precedents regarding publicly accessible data.
Best Practices for Responsible Scraping
To navigate these challenges ethically:
- Implement reasonable rate limiting (1 request every 2-5 seconds)
- Consider using Amazon’s official API where available
- Scrape only the data you genuinely need
- Respect robots.txt directives
- Store data securely and in compliance with relevant privacy laws
By addressing these challenges with appropriate technical solutions and ethical considerations, developers can create sustainable Amazon scraping systems that provide valuable data while minimizing risks.
Essential Tools and Libraries
Successfully scraping Amazon requires the right set of Python tools and libraries. This section explores the most effective options available in 2025, comparing their features, benefits, and ideal use cases.
Core Python Libraries for Amazon Scraping
Library | Description | Best For |
---|---|---|
Requests | Simple HTTP library for making web requests | Basic scraping of static pages |
Beautiful Soup | HTML/XML parsing library | Extracting data from HTML responses |
Scrapy | Comprehensive web crawling framework | Large-scale, production scraping projects |
Selenium | Browser automation tool | Handling JavaScript-rendered content |
Playwright | Modern browser automation library | Stealth scraping with advanced capabilities |
HTTPX | Async HTTP client | Concurrent requests with modern Python |
Proxy and IP Rotation Services
For serious Amazon scraping projects, proxy services are nearly essential:
- Bright Data: Enterprise-grade proxy infrastructure with specific e-commerce scraping capabilities
- ScraperAPI: API that handles proxy rotation and CAPTCHA solving
- Oxylabs: E-commerce-specific proxy solutions with built-in parsing features
- Zyte (formerly Scrapinghub): End-to-end scraping solutions with smart proxy management
CAPTCHA Handling Solutions
As Amazon’s CAPTCHA systems have grown more sophisticated, specialized tools have emerged:
- 2Captcha/Anti-Captcha: Human-powered CAPTCHA solving services
- undetected-chromedriver: Modified Selenium driver that bypasses detection
- Playwright Stealth: Browser automation with enhanced fingerprint masking
Data Processing and Analysis Tools
Once data is collected, these libraries help transform raw information into actionable insights:
- Pandas: Data manipulation and analysis
- NLTK/spaCy: Natural language processing for review analysis
- Matplotlib/Seaborn: Data visualization
- SQLAlchemy: Database integration for storing scraped data
The optimal toolset for scraping Amazon depends on your specific requirements, technical expertise, and project scale. For beginners, a combination of Requests and Beautiful Soup provides a gentle entry point. For production-grade applications, Scrapy with proxy rotation services offers the reliability and scalability needed for ongoing data collection.
Advanced Scraping Techniques
Mastering scraping Amazon requires going beyond basic approaches to implement advanced techniques that enhance reliability, efficiency, and stealth. This section explores sophisticated strategies that experienced developers use to overcome Amazon’s increasingly complex anti-scraping measures.
Browser Fingerprint Management
Modern anti-bot systems analyze browser fingerprints—unique combinations of settings, extensions, and capabilities that can reveal automated tools. Advanced Amazon scrapers modify these fingerprints to appear more human-like:
# Example of browser fingerprint management with Playwright
from playwright.sync_api import sync_playwright
def configure_stealth_browser():
with sync_playwright() as p:
browser = p.chromium.launch(
headless=True,
args=[
'--disable-blink-features=AutomationControlled',
'--user-agent=Mozilla/5.0 (Windows NT 10.0; Win64; x64) AppleWebKit/537.36 (KHTML, like Gecko) Chrome/110.0.0.0 Safari/537.36',
]
)
context = browser.new_context(
viewport={'width': 1920, 'height': 1080},
user_agent='Mozilla/5.0 (Windows NT 10.0; Win64; x64) AppleWebKit/537.36 (KHTML, like Gecko) Chrome/110.0.0.0 Safari/537.36',
geolocation={'longitude': -122.0, 'latitude': 37.7},
locale='en-US',
)
# Disable webdriver flag
page = context.new_page()
page.add_init_script("""
Object.defineProperty(navigator, 'webdriver', {
get: () => false
});
""")
return browser, context, page
Intelligent Rate Limiting
Rather than using fixed delays between requests, advanced scrapers implement dynamic rate limiting that adjusts based on server response times and patterns:
- Exponential Backoff: Increasing wait times when encountering resistance
- Time-of-Day Adaptation: Adjusting scraping intensity based on Amazon’s traffic patterns
- Response-Time Monitoring: Slowing down when server responses indicate potential throttling
Distributed Scraping Architecture
Enterprise-level Amazon scraping often employs distributed systems that spread requests across multiple machines, IP ranges, and geographic locations. This approach significantly reduces detection risk while increasing throughput:
- Job Queues: Using RabbitMQ or Redis to distribute scraping tasks
- Microservice Architecture: Separating proxy management, request handling, and data processing
- Geographic Distribution: Running scrapers from different global regions to match target market locations
Machine Learning for Pattern Recognition
Some advanced systems now incorporate machine learning to identify patterns in Amazon’s page structure, allowing for more resilient data extraction when elements change:
- Visual Recognition: Identifying product information based on visual layout rather than HTML structure
- Adaptive Selectors: Learning and adjusting CSS/XPath selectors based on success rates
- Anomaly Detection: Identifying unusual responses that might indicate anti-scraping measures
Session Management and Cookies
Maintaining consistent sessions with proper cookie handling significantly improves scraping success rates:
# Example of session management for Amazon scraping
import requests
from http.cookiejar import MozillaCookieJar
class AmazonScraper:
def __init__(self):
self.session = requests.Session()
self.cookiejar = MozillaCookieJar('amazon_cookies.txt')
# Try to load existing cookies
try:
self.cookiejar.load()
self.session.cookies.update(self.cookiejar)
except FileNotFoundError:
pass
self.session.headers.update({
'User-Agent': 'Mozilla/5.0 (Windows NT 10.0; Win64; x64) AppleWebKit/537.36 (KHTML, like Gecko) Chrome/110.0.0.0 Safari/537.36',
'Accept': 'text/html,application/xhtml+xml,application/xml;q=0.9,image/webp,image/apng,*/*;q=0.8',
'Accept-Language': 'en-US,en;q=0.9',
'Accept-Encoding': 'gzip, deflate, br',
'Connection': 'keep-alive',
'Upgrade-Insecure-Requests': '1',
'Sec-Fetch-Dest': 'document',
'Sec-Fetch-Mode': 'navigate',
'Sec-Fetch-Site': 'none',
'Sec-Fetch-User': '?1',
'DNT': '1'
})
def save_cookies(self):
# Transfer current cookies to cookiejar
for cookie in self.session.cookies:
self.cookiejar.set_cookie(cookie)
self.cookiejar.save()
These advanced techniques represent the cutting edge of Amazon scraping in 2025. While implementing them requires greater technical expertise, they dramatically improve success rates and data quality, especially for large-scale or ongoing scraping operations.
Case Study: Price Monitoring System
To illustrate the practical application of scraping Amazon, let’s examine a real-world implementation of a price monitoring system built with Python. This case study demonstrates how the concepts and techniques discussed throughout this guide come together in a functional application.
Project Background
An e-commerce retailer needed to track competitor pricing across 500+ products on Amazon to maintain competitive pricing while preserving profit margins. Manual monitoring was impossible given the volume of products and frequency of price changes.
System Architecture
The solution implemented a three-tier architecture:
- Data Collection Layer: Scrapy-based scrapers with proxy rotation
- Data Processing Layer: Pipeline for cleaning, normalizing, and analyzing price data
- Reporting Layer: Dashboard and alert system for price changes
Core Implementation
# Simplified example of the Amazon price scraper
import scrapy
from scrapy.crawler import CrawlerProcess
from itemadapter import ItemAdapter
import logging
import json
from datetime import datetime
class AmazonPriceItem(scrapy.Item):
product_id = scrapy.Field()
asin = scrapy.Field()
title = scrapy.Field()
price = scrapy.Field()
currency = scrapy.Field()
url = scrapy.Field()
timestamp = scrapy.Field()
class AmazonPriceSpider(scrapy.Spider):
name = 'amazon_price_monitor'
def __init__(self, product_list='products.json', *args, **kwargs):
super(AmazonPriceSpider, self).__init__(*args, **kwargs)
# Load product list from JSON file
with open(product_list) as f:
self.products = json.load(f)
def start_requests(self):
for product in self.products:
url = f"https://www.amazon.com/dp/{product['asin']}"
yield scrapy.Request(
url=url,
callback=self.parse_product,
meta={'product_id': product['internal_id'], 'asin': product['asin']}
)
def parse_product(self, response):
# Extract price using multiple selector patterns for resilience
price_selectors = [
'//span[@id="priceblock_ourprice"]/text()',
'//span[@id="priceblock_dealprice"]/text()',
'//span[contains(@class, "a-price-whole")]/text()'
]
price = None
for selector in price_selectors:
price_raw = response.xpath(selector).get()
if price_raw:
# Clean up price text
price = ''.join(filter(lambda x: x.isdigit() or x == '.', price_raw))
try:
price = float(price)
break
except ValueError:
continue
title = response.xpath('//span[@id="productTitle"]/text()').get()
if title:
title = title.strip()
# Create item only if we successfully extracted price
if price:
item = AmazonPriceItem()
item['product_id'] = response.meta['product_id']
item['asin'] = response.meta['asin']
item['title'] = title
item['price'] = price
item['currency'] = 'USD' # Assuming USD for simplicity
item['url'] = response.url
item['timestamp'] = datetime.now().isoformat()
yield item
else:
logging.warning(f"Failed to extract price for ASIN: {response.meta['asin']}")
# Pipeline for price change detection
class PriceAlertPipeline:
def __init__(self):
self.previous_prices = {}
# Load previous prices from database in real implementation
def process_item(self, item, spider):
adapter = ItemAdapter(item)
product_id = adapter.get('product_id')
current_price = adapter.get('price')
if product_id in self.previous_prices:
prev_price = self.previous_prices[product_id]
price_diff = current_price - prev_price
price_change_pct = (price_diff / prev_price) * 100
# Alert on significant price changes
if abs(price_change_pct) > 5: # 5% threshold
logging.info(f"Price alert: {adapter.get('title')} changed by {price_change_pct:.2f}%")
# In real implementation, send email/notification here
# Update price record
self.previous_prices[product_id] = current_price
return item
# Run the spider if executed directly
if __name__ == '__main__':
process = CrawlerProcess({
'USER_AGENT': 'Mozilla/5.0 (Windows NT 10.0; Win64; x64) AppleWebKit/537.36 (KHTML, like Gecko) Chrome/110.0.0.0 Safari/537.36',
'ROBOTSTXT_OBEY': True,
'DOWNLOAD_DELAY': 3, # 3 seconds between requests
'CONCURRENT_REQUESTS': 1,
'ITEM_PIPELINES': {
'price_monitor.PriceAlertPipeline': 300,
}
})
process.crawl(AmazonPriceSpider)
process.start()
Implementation Challenges and Solutions
During development, the team encountered several challenges:
- Challenge: Frequent IP blocking
Solution: Implemented rotating residential proxies with 200+ IPs - Challenge: Varying price selector patterns
Solution: Used multiple fallback selectors and regular expression patterns - Challenge: High failure rate during peak hours
Solution: Implemented time-of-day scheduling to focus on off-peak hours
Results and Business Impact
After six months of operation, the system delivered significant business value:
- Price monitoring coverage increased from 30% to 98% of competitor products
- Average response time to competitor price changes decreased from 32 hours to 3 hours
- Profit margins improved by 12% through smarter pricing decisions
- Development cost was recouped within 4 months through improved pricing strategy
This case study demonstrates how scraping Amazon with Python can deliver tangible business value when implemented thoughtfully. The combination of robust technical design, error handling, and business integration created a system that significantly improved decision-making capabilities.
Frequently Asked Questions
Is scraping Amazon legal?
Frequently Asked Questions
Is scraping Amazon legal?
Scraping Amazon involves navigating complex legal considerations. Amazon’s Terms of Service explicitly prohibit automated data collection without permission, and violating these terms could lead to account suspension or legal action. However, the legality of web scraping public data remains a gray area, influenced by cases like hiQ Labs v. LinkedIn, which suggested that scraping publicly accessible data may not violate computer access laws in some jurisdictions. Always consult a legal professional to ensure compliance with local laws, Amazon’s terms, and regulations like GDPR or CCPA when handling personal data.
What are the best Python libraries for scraping Amazon?
The best Python libraries for scraping Amazon in 2025 include:
- Scrapy: Ideal for large-scale, production-grade scraping projects.
- Beautiful Soup: Perfect for parsing HTML and extracting data from static pages.
- Selenium/Playwright: Essential for handling JavaScript-rendered content and CAPTCHAs.
- Requests/HTTPX: Great for making HTTP requests, with HTTPX supporting asynchronous operations.
Choose based on your project’s scale and complexity. For example, combine Scrapy with Playwright for robust, stealthy scraping.
How can I avoid getting blocked while scraping Amazon?
To avoid detection and blocking by Amazon’s anti-scraping measures, consider these strategies:
- Use Proxy Rotation: Services like Bright Data or Oxylabs provide rotating IPs to prevent rate limiting.
- Implement Stealth Techniques: Use Playwright with stealth plugins or undetected-chromedriver to mask automation fingerprints.
- Respect Rate Limits: Space requests (e.g., 1 request every 2-5 seconds) and use random delays to mimic human behavior.
- Manage Cookies: Maintain session consistency to avoid triggering CAPTCHAs.
- Monitor Responses: Dynamically adjust scraping patterns if you detect throttling or CAPTCHA challenges.
Can I use Amazon’s official API instead of scraping?
Yes, Amazon provides the Amazon Product Advertising API, which allows access to product data, prices, and reviews for authorized developers. This is a legal and preferred alternative to scraping, though it has limitations, such as request quotas and restricted data fields. To use the API, you need an Amazon Associate account and must comply with its terms. If the API meets your needs, it’s a safer and more sustainable option than scraping. For details, visit Amazon’s API documentation.
How can I handle CAPTCHAs when scraping Amazon?
Handling CAPTCHAs requires a combination of prevention and resolution strategies:
- Prevent CAPTCHAs: Use stealth browsers (e.g., Playwright with stealth mode), rotate IPs, and mimic human-like request patterns.
- Resolve CAPTCHAs: Integrate services like 2Captcha or Anti-Captcha, which use human solvers or AI to bypass CAPTCHAs.
- Automate Detection: Build logic to detect CAPTCHA pages and pause scraping or switch IPs when encountered.
Be cautious, as frequent CAPTCHA triggers may indicate overly aggressive scraping, increasing the risk of IP bans.
What data can I scrape from Amazon?
You can scrape various publicly available data from Amazon, including:
- Product Details: Titles, descriptions, ASINs, and categories.
- Pricing Information: Current prices, discounts, and historical price trends.
- Customer Reviews: Ratings, comments, and reviewer metadata (anonymized to comply with privacy laws).
- Seller Information: Seller names, ratings, and fulfillment details (e.g., FBA or FBM).
- Best Seller Rankings: Category rankings and trending products.
Always ensure your use case complies with legal and ethical guidelines, and avoid scraping protected content like copyrighted images or personal data.
Conclusion
Scraping Amazon with Python in 2025 is a powerful strategy for businesses and professionals seeking to harness the wealth of data available on the world’s largest e-commerce platform. From competitive pricing to product research and sentiment analysis, the applications of Amazon scraping are vast and transformative. However, success requires navigating technical challenges, such as anti-scraping measures, and adhering to legal and ethical boundaries.
This guide has provided a comprehensive roadmap, covering the significance of Amazon scraping, its evolution, practical applications, tools, advanced techniques, and real-world case studies. By leveraging Python’s robust ecosystem—libraries like Scrapy, Playwright, and Beautiful Soup, alongside proxy services and CAPTCHA solutions—you can build scalable, efficient, and responsible scraping systems.
As Amazon continues to evolve its platform and anti-scraping technologies, staying informed about best practices and emerging tools will be critical. Whether you’re a developer, e-commerce entrepreneur, or market researcher, mastering Amazon scraping equips you with the insights needed to thrive in a competitive marketplace.
For those looking to get started, consider exploring Amazon’s official API as a legal alternative, and always prioritize ethical scraping practices to ensure sustainability. With the right approach, scraping Amazon can unlock unparalleled opportunities for data-driven decision-making in 2025 and beyond.
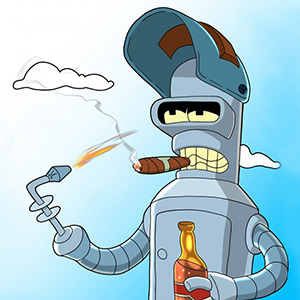
Professional data parsing via ZennoPoster, Python, creating browser and keyboard automation scripts. SEO-promotion and website creation: from a business card site to a full-fledged portal.